How Quality Data Annotation Services Improve the Performance of Autonomous Vehicles 11 Nov 2024
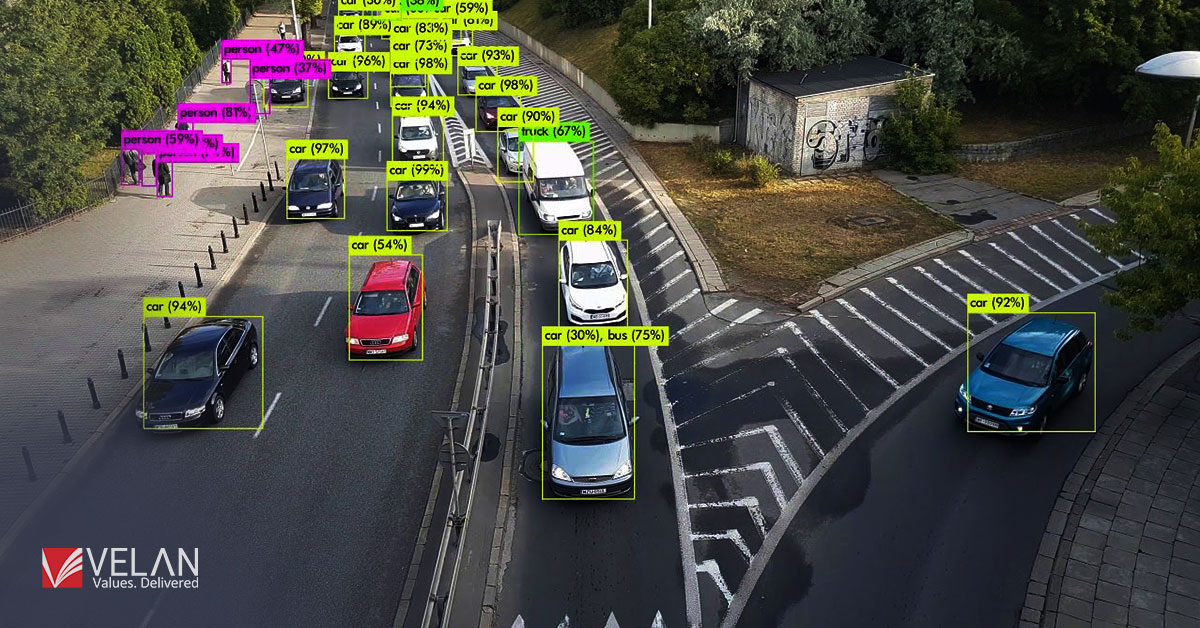
It is armed with databases of annotated data that “teach” AV systems to visually process and understand the world, which in turn lies at the core of what will one day power incredible autonomous vehicles (AVs). A new report from Allied Market Research forecasts the global autonomous vehicles market will reach $556. It continues to highlight the rise of precision data annotation services.
Development of AI in Autonomous Vehicles: Data Annotation The result is super precise sensing and perception for autonomous vehicles. Optimal performance and safety of this annotated data thus tend to be enhanced by these vehicles.
This ensures the accurate detection of road status, obstacles, and objects. A high level of data annotation is the clear-cut transformation from raw information to a valuable one. It helps to make autonomous decisions in self-driving cars with real-time confidence.
In this blog, we’ll look into the function of data annotation and how it can help enhance AV functionality with autonomous driving solutions serving as secure yet efficient commuting alternatives.
Role of Data Annotation in Enhancing the Efficiency of Self-Driving Cars
Top-tier data annotation converts raw data into labeled datasets that machine learning models can learn from and improve. This annotated data enhances functions in AV systems such as object detection and classification, lane and road sign recognition, pedestrian and obstacle detection, or environment perception and context comprehension from various sensors.
Detection and Recognition Objects
Accurate object detection and classification is important for a vehicle to navigate safely in autonomous mode. Training AV algorithms on annotated datasets enables these vehicles with the ability to efficiently detect and classify objects. This helps inform driving decisions, prevent crashes, and protect pedestrians as well as occupants.
Detection of Road and Lane Markings
With annotated data, lane markings and road signs become more distinguishable in the face of poor road quality, changing weather conditions, and low illumination. The New AVs are taught to perceive lanes and signs accurately with the help of annotated images as well as videos. It helps them stay in their lane, change lanes, and obey traffic signals and speed limits.
Obstacle and pedestrian detection.
This includes AV systems predicting pedestrian behaviour, differentiating between stationary and moving objects within a scene or environment, and taking appropriate vehicle actions as the result of those predictions. This means adding labels to different pedestrian poses, movements, and positions of the auto.
Context Sensitive and Ambient Intelligence
It will enable the AVs to read roads, recognize potential obstacles, and predict what other users will do. Training the AV models on annotated datasets allows them to have more extensive situational analysis and make driving decisions that are not only precise but predictive as well.
Data Annotation Techniques in Autonomous Cars
Self-driving cars (AVs) have a sixth sense, which is no more magical than carefully labeled data. That’s exactly what this data annotation does, breathing new life into sensor data and allowing vehicles powered with autonomous capabilities (Autonomous Vehicles—AVs) to navigate through the complexities of the road.
Some critical methodologies include the following types, which are only given a short introduction here in cosmology.
Radar and LiDAR Annotation
3D point cloud data contains points for human specialists to label objects (i.e., pedestrians, vehicles, and obstacles). As a result, AVs can get an accurate understanding of what is around them, especially in low-light situations or inclement weather, which are tricky conditions for cameras.
Instance-level and semantic segmentation
Images have semantic segmentation for each pixel. It identifies the road, sidewalk, car, person, or anything related. Such an exhaustive analysis aspect gives AV a complete understanding of the scenario. This is complemented by instance segmentation capabilities that can help an AV detect a particular car or a pedestrian instead of treating all instances within the class similarly.
Annotation of Sensor Fusion
It provides a common dataset that looks at the environment as a whole. An AV is able to make better decisions and have a holistic view of complex situations by combining elements of all senses.
Bounding Boxes
Think of a box that has been drawn around parts and pieces in an image like this impartial pedestrian or vehicle. You see this a lot in object detection (bounding box). It guides AVs on how to find different objects on or off-road.
Cuboids in three dimensions
Autonomous vehicles must really sense the real 3D space. 3D cuboids localize objects in 2 dimensions with width and height and get depth information. This enables the accurate calculation of distances and navigation in complex environments for autonomous vehicles.
Finding the Right Data Annotation Partner for Autonomous Vehicles
The perfect performance of your self-driving car initiatives has to do with choosing and hiring an ideal data annotation partner for autonomous cars. With proper data annotation, machine learning models can be trained to accurately interpret and move around their surroundings.
First, look for a used car data provider who has expertise in the automotive industry and can handle big datasets. They also need to have the necessary technological background and enough annotators that are well-taught to meet the needs of your project.
Second, evaluate what systems of quality assurance currently exist. Ensure that the partner has an extensive quality assurance system built-in, which comprises basic AI checks and several stages of assessment and validation from expert annotators. This helps to reduce errors as well as improve consistency in a larger data set.
Naturally, your partner must also adhere to strict security measures and protect the data from vulnerabilities or authorized access.
Ultimately, partner with someone who can accommodate your ever-changing needs and customize answers while being flexible.
What lies ahead with data annotation for autonomous vehicles?
Data annotation is expected to greatly impact the evolution of autonomous vehicle (AV) technology for years to come. These trends and innovations will help improve the efficiency of AV systems, revolutionizing various components within an industry.
Automation Boosts: Automation greatly speeds the processing of such large amounts of data, which is transforming how annotations are done. This makes current, balanced training datasets more accessible to the AV-Bio minority models and helps speed their development.
Higher Quality Control: By following state-of-the-art quality control standards like agreeing-based annotations and real-time error fixation, we shall ensure that no transcription or annotation goes through poor specification. This will make error files more reliable across different AV systems.
Target time: New platforms will allow annotators, AI researchers, and AV developers to work together in real time. This will enrich the content of annotations and stimulate a single data annotation ecosystem.
Ethical and Privacy Concerns: As things move more towards labeling in a real-world setting, we will also need to improve our ability to address privacy considerations from day one. Eventually, secure data management approaches and privacy precautions become must-have best practices.
Integration with Simulation Environments: It will be to combine labeled real-world data and generated synthetic simulations into the same training dataset. This will allow AV systems to learn more quickly and adapt/operate in new scenarios.
Continuous Learning and Adaptation: In the future, annotation will be an ongoing process of updating AV datasets to maintain model accuracy as new data is generated in real-time. This form of lifelong learning is vital to ensuring AVs remain safe and efficient.
Benefits of Quality Data Annotation in Autonomous Vehicles
Quality data annotation is a must-have in both AV development and operation. It transforms unstructured data into structured, labeled datasets that enable machine learning models to “understand” and react correctly to the world around them. Here are the key benefits to top-tier data annotation for autonomous vehicles:
Better Object Recognition: AVs can detect and categorize items on the road like cars, people, bikes, or cones with better annotation accuracy. The AVs can then react accordingly to avoid those collisions and ensure an intuitive driving experience.
Increased Situational Awareness: AVs gain a more comprehensive understanding of their environment, such as the weather conditions, lane markings, and road signs. This allows them to drive better—for instance, slow down when the roads are slippery and follow traffic regulations.
Improved Decision-Making Under Critical Conditions: AVs encounter unexpected situations. This becomes critical only when good-quality data annotations are available for labeling of inputs. By annotating data for rare occurrences such as unexpected pedestrian gestures or inclement weather, AVs can be taught to respond accordingly and mitigate associated risks.
Versatility on a High Spectrum: Automated cars face multiple types of road conditions, and autonomous is equipped to deal with all of them. They can deal with it by quality data annotation. By relying on input from multiple locations and weather conditions, AVs can drive assuredly anywhere and adapt their behaviour to the full range of illumination situations.
Improved Safety and Reliability: The end goal of autonomous vehicle design is to create secure, reliable self-driving cars. Quality training data enable this future by providing quality annotation. Through its adeptness in handling adversarial events, heightened situational awareness, and sharp object recognition, it enhances the safety of all road users.
In conclusion,
The quality of the data annotation in turn plays a key role towards developing quiet and reliable autonomous vehicles. AI-driven autonomous vehicles can make safe decisions to safely manoeuvre through such multi-faceted ecosystems and across them by being trained with accurately annotated data. Data annotation methods are advancing, which means that self-driving cars will be even more qualified over time.
Recognizing the imperative contribution of data annotation for AI profitably at Velan, powered by a global community of expert annotators, we offer a full spectrum suite of data annotation services. We know how to consult in many industries and data types, which allows for 100% customization of methodology