Unlocking AI Potential: The Essential Role of Data Annotation in Machine Learning 23 Oct 2024
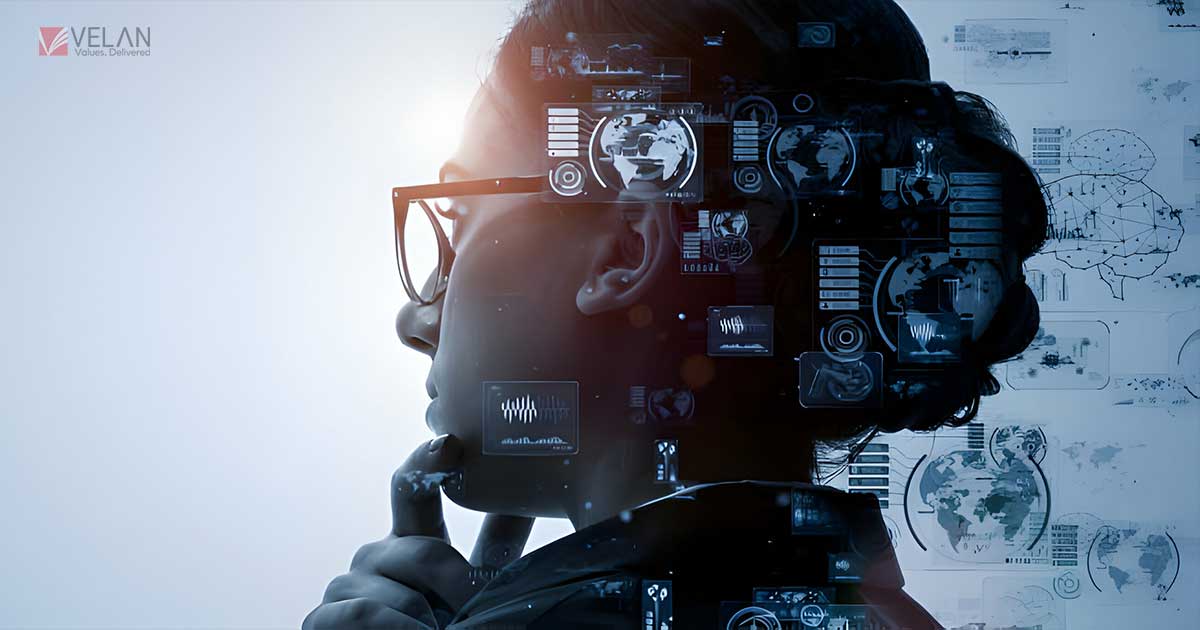
How can we overlook artificial intelligence (AI) and machine learning (MML) in our fast-paced, technology-driven modern life? Artificial intelligence (AI) is utilizing amazing new technologies to transform our lives, from smart home devices and self-driving cars to your favorite TV show streaming service or GPS that anticipates traffic. Artificial intelligence (AI) has the potential to add $13 trillion in global economic activity by 2030, making it one of the most critical technologies that is capable of transforming industries and societies across all geographies. Unlocking the real power of AI, however, depends heavily on a step that is both critical and commonly ignored—data annotation. Properly labelled, high-quality training data forms the foundation of any successful machine learning model, enabling AI systems to learn from accurate information and adapt to future circumstances. This is where the importance of data annotation in machine learning comes into play, and we will look deep down at what impact it has.
AI and ML models require a substantial amount of training data to achieve all of this. Computers are not capable of processing visual information in the same way people see. To make decisions, computers must be able to define and sense the data they are interpreting. Data annotation for machine learning builds these links.
What does data annotation mean in machine learning?
Only because of data annotations can machine learning models recognize patterns, draw assumptions, and carry out complex tasks. Annotated or labelled datasets make sure training models can appropriately interpret and classify new, never-before-seen data in the same way as humans do.
How does work on data annotation in machine learning?
In the simplest of terms, data annotation involves labelling datasets with tags that lend context and meaning (and, as a result, key information) to enable algorithms to recognize patterns for accurate predictions. At the very beginning of this process, raw data, which may include images, text, and audio (in a nutshell, everything there is), are collected. After that, annotators tag or mark the data with relevant details, such as sentiment tags in the case of text and bounding boxes for object detection in images.
In the absence of human-annotated datasets, AI and ML algorithms fail to accurately calculate these key features.
Pros of Data Annotation for AI and ML Companies
Artificial and machine learning platforms are currently being used almost unrestrictedly across a wide range of applications. However, a thick layer of hype and fanciful approaches envelop AI/ML, masking the challenges and numerous obstacles that companies offering these platforms encounter when working with precisely annotated training data. Superior-quality training datasets— The success of AI and ML projects rely heavily on the accuracy of annotated data. AI/ML companies need well-annotated datasets to train generalized AI models amenable to identifying the patterns and relationships of multiple variables. Analytics companies cannot afford misaligned bounding boxes and confusion in the classifiers. This implies that the accuracy of data curation and labels is necessary to ensure that AI/ML models are personalized and optimized for efficiency.
It’s all about the data: AI and ML models are data-hungry—most successful ML projects require tens of thousands, or in some cases, millions, of labeled training objects. Machine learning projects can be extremely complex, but they all need good-quality data to train their model.
According to the McKinsey Global Institute, 75% of AI and ML projects need monthly updates on learning datasets, while 24% rely on daily improvements in annotated data sets.
Data annotation projects: AI & ML companies face a shortage of manpower to handle complex and large data annotation processes. Reassigning engineers or other staff from their main tasks to do data labeling is an expensive process. Without a continuous flow of accurately labeled data, AI and ML companies struggle to create models that can accurately interpret important features or make accurate predictions.
This is one of the reasons why we are witnessing massive growth in global market size, from US $695.5 million back in 2019 to a projected rise to US $6,450.0 million by 2027—big surprise, right?
Data labeling in AI and ML models offers the following edges:
Text annotation, image annotation, or video sports analytics help us provide a better understanding of the meanings of the text or objects, thus making it easier for algorithms to perform at their best.
AI and ML models have become even more accurate.
When detecting multiple objects in an image, a computer vision model operates partially and poorly between two extremes: one where all objects receive correct labeling, and another where no labeling occurs at all. Therefore, the precision of the model increases as the quality of the annotation increases.
Faster model training
We successfully reduced the time-to-approach (TAT) for a data analysis services provider’s machine learning initiative by 54%. A data annotation company analyzed the traffic signal footage to recognize vehicles and classify them according to their type, model name, color, as well as direction. Only with this cautiously annotated database can an effective AI and ML model understand what actions are required to manage the data it receives. Thus, the model quickly learns how to properly treat the train data and gives interpretable results.
Easy generation of labeled datasets
Adding annotations is a crucial step in building your machine learning dataset, as it significantly accelerates the preprocessing process. An example of this occurred in labeling and feeding, manually via human workers and programmatically by machine workflows such as loading 40K+ images into various ML models. It helped a Swiss-based food processing company reduce the massive waste in leading hotels and restaurants. This is where the regularization of data annotation services leads to a proliferation of labeled datasets and AI and ML models that assume full functional support.
A streamlined end-user experience
Well-annotated data provides a smooth journey for the consumers of AI systems. Intelligent products that are smart, functional, and in context effectively address user questions and doubts, thereby facilitating motive support. Relevance protection is enabled by annotation.
Strengthening foundations for AI engines through progressive
These assumptions assume that more data improves AI model accuracy and precision, but only if there is labeled data. Therefore, we expect the AI engines to increase in reliability as the data scales.
Makes it possible to scale deployments
Annotated data can easily incorporate the sentiment, intent (target), and action of thousands of requests. Furthermore, it facilitates the creation of high-accuracy training datasets, uniquely allowing AI experts and data scientists to scale mathematical models across any size or type of dataset.
Data annotation and labeling can be broken down into four general categories:
Once more, the process of data annotation for machine learning encompasses all aspects and follows a series of steps to determine the types of annotations that can be available. The most commonly used types of annotations are,
-
Text annotation
Search engines commonly employ text annotations, which annotate words to facilitate the engine’s algorithms when you click on keywords, enabling the loading of pages containing those keywords. Search engines quickly generate relevant results by mapping tags to videos and URLs across databases. Below is a more pragmatic point of view.
-
Video annotations
Autonomous vehicles are among the numerous instances where video annotation is essential, yet they are the most frequently featured in blogs. Technically, it splits video into frames with a categorical object label(s). Thus, the video annotations deliver a substantial on-road safety enhancement by offering an exhaustive investigation of road traffic dynamics as well as in-cabin driver milestones and regions prone to accidents.
A California-based data analytics company hired an outsourced data annotation firm to annotate the predefined categories on live streaming and pre-recorded video streams for ML models. This enabled them to promptly implement a dashboard that displays directional traffic volumes, complete with real-time data and alerts for city-level snapshots. The dashboard also shared the number of vehicles collected per hour, based on historical volumes.
-
Annotations to images
On the heels of 200 million highly annotated images, we enabled search experiences that local customers and global partners alike could experience in markets throughout the US and abroad for the world’s largest technology company. With a highly reliable training dataset, users were able to find images that, while appropriate for the given search query intent, were also free of spam in real time.
Annotations are various techniques that are used to define regions of concern in an image, such as bounding boxes, polygons, tracking, and masking, among others. Machine learning specialists pre-specify facets to give the computer vision models essential information. One or more of these object detection techniques can label objects in an image, depending on the context.
-
NLP Corps for Speech Recognition Annotation
This activity, designed to train a virtual assistant for optimal understanding and response to a minimum of 150 million active monthly users, required the transposition of complex grammar rules into each of the 14 languages he served, along with quality checks over pronunciations and corrected transcriptions.
Because of the nature of our human-made labeling, NLP annotation primarily focuses on language and extracts unique insights. The tool encompasses various NLP annotation processes, including POS tagging, phonetic annotation, semantic annotation, key term tagging, and discourse annotation, all of which contribute to capturing the unique characteristics of language construction. It helps machine learning understand the context and meaning of things, just as humans do.
-
A new age of data tagging is on the horizon
Grand View Research estimates that the global data annotation market will grow up to 1.6 US billion in the next four years, and another market report by Research & Markets anticipates it will reach 6.45 US billion by 2027.
In conclusion, these technological advancements in space are expected to significantly boost the growth of the data annotation market.
The future of artificial intelligence and machine learning is smart labeling tools. All data labeling capabilities will be fully automated, utilizing predictive analytics to identify labels without human intervention.
The process of data annotation will also involve the use of reporting frameworks. Operational intelligence can aid in comprehending the solutions to annotation challenges. The reporting functionalities will undoubtedly aid in monitoring and managing the throughput and productivity of annotations.
Automation and quality assurance are necessary to annotate data at scale accurately (more on this later). This will serve as a vital determinant of future data annotation, orienting labeling toward quality and measured marking rather than just mere tagging.
Annotation or labeling services have broadened machine learning projects to a large extent. It incorporates vetted workflows, annotation tools, and expert human annotators to produce massive volumes of training and testing data that are clean and properly labeled!
Velan provides world-class data annotation services. We provide the highest quality and most accurate labeled datasets for your AI projects. These are some of the ways we tailor our data annotation services to enhance AI and machine learning efforts. We consistently stay updated with the latest industry features to ensure you receive crisp and precise annotations for your AI initiatives. Your AI models have to work properly and deliver results in the field, which is what our accurate data labeling does.
In conclusion,
This combination of human intelligence and smart tools is the ideal approach for data annotation, as it aids in the creation of effective training datasets that serve as feeds for machine learning models. So, companies must develop strong data annotation abilities to reduce the risks of AI and ML model failures. This illustrates the superiority of humans over computers in handling ambiguity, deducing intended meaning, and taking into account various other factors that influence the intent-based annotation of text, video, or images.
The future of a high-performing AI/ML model that correctly answers even the most complex business question or an operation on which someone has spent months entirely depends upon how well data and annotated are trained. If a lack of time and resources prevents you from developing these skills, it would be beneficial to hire data annotation companies. Not only do specialists optimize your time and money, but they also provide a faster path to enhanced AI capabilities that effectively address market needs and attract the diverse customer base you desire.
We at Velan comprehend the vital role of data annotation in the success of AI. Our global network of expert annotators and state-of-the-art technologies support our comprehensive array of data annotation solutions. Our team’s proficiency in a variety of industries and data types enables us to customize our approach to meet your requirements.